Intro to Networks
Network theory is a mathematical framework that studies the connections (edges), between elements (nodes) that can define regions (areas) within a network. This concept can be applied to various real-world systems, including basketball. In the context of basketball, players can be considered as nodes, and their interactions and connections on the court can be represented as edges, that can divide the court itself into regions (zones).

In basketball, connectivity refers to the players' ability to establish passing lanes, create scoring opportunities, and maintain a coherent defensive structure. By analyzing the flow of passes, player movements, and decision-making on the court, we can gain valuable insights into the dynamics of the team's network. Understanding the team's ability to space the floor, communicate, as well as read and react to each other's movements greatly enhances the overall connectivity and increases the chances for victory.
There are different types of networks:
• An undirected network considers equal connections between nodes.
• A directed network acknowledges the presence of directed edges. In basketball, there is a degree of directionality as you would expect the meaningful part of a ball movement sequence to start with the point guard, but no flow is solely unidirectional.
By applying network science to basketball, analysts can enhance traditional basketball statistics by considering individuals in relation to their teammates and competitors. Instead of analyzing players independently, network-based analytics construct a team or league-level player through their connections. By calculating various network properties such as degree, density, centrality, clustering, and distance, analysts can obtain new individual and team-level statistics. This approach provides a fresh perspective on player positions, as it assigns positions based on network dynamics.
There are several network measures:
-
The team clustering coefficient indicates the interconnectedness of players and whether the ball moves through a single node or among all players in various ways.
-
Analyzing network centralization can help determine whether a team relies too heavily on an individual player or if the workload is effectively distributed. Teams with a combination of low clustering and high degree centrality suggest the defense can double-team the dominant player.
-
Basketball players need to strategically navigate the court to find open spaces, cut to the basket, or set screens for their teammates. Analyzing optimal pathfinding can reveal patterns, offensive strategies, and defensive rotations that lead to higher success rates. A subset of pathfinding is the uphill downhill flux metric, which measures the division of labor in moving the ball to the player with the best shooting percentage.
-
The average path length statistic represents the number of passes per play, providing insights into the team's passing efficiency.
-
Resilience measures a network's ability to maintain functionality and performance despite external disturbances or the removal of specific nodes. In basketball, resilience refers to a team's adaptability to different game situations, ability to overcome injuries or fouls, and capacity to adjust strategies on the fly. Analyzing resilience can pinpoint areas where a team needs to improve its adaptability and develop contingency plans.
-
Team entropy measures the unpredictability and variation in a team's offense by assessing the uncertainty of ball movement between any two nodes.
-
Geographically, networks can incorporate varying types of nodes and edges. For example, colored nodes and different line styles can represent distinct node and edge types, respectively. Weighted graphs can also be utilized to capture the differences among nodes and edges, where the size of nodes and edges signifies the strength of their interactions.

*Note: Here, the Regions are colored in. Most network diagrams are not usually colored in.
There are four Areas or “Regions” on the above diagram, because the outside Blue area is also counted as a Region.
There are five “Vertices” on the diagram which connect together the lines and curved edges.
The above Network has seven “Edges”. (Note that Edges can be straight lines or curves).
Network Equation: There is an equation which works for every Network, and is useful for checking answers.
The Equation is: Regions + Vertices = Edges + 2
In our sample Network shown previously, substituting into the above Equation gives:
4 + 5 = 7 + 2
If the equation does not work out equal on both sides for a Network, then one of our counts of the Vertices, Edges, or Regions must be incorrect.
Not all nodes and edges are created equal. To model such difference, one can introduce different types of nodes and edges in the network, as illustrated by the different colors and edge styles, above. In networks where the differences among nodes and edges can be captured by a single number that, for example, indicates the strength of the interaction, a good model may be a weighted graph. One can represent a weighted graph by different sizes of nodes and edges.

An undirected network, where the nodes and edges have different types, as indicated by their colors and line styles.

A directed network, where the edges and nodes have different weights, as indicated by their sizes.
Applying network science to basketball enables analysts to gain a more comprehensive understanding of the game. It allows for a holistic assessment of player dynamics, team connectivity, offensive and defensive strategies, and overall team performance.
STEM Topic 26: VOCABULARY
Allocate: To distribute or assign for a particular purpose.
Anarchy: A state of disorder or chaos, where there is no authority or control.
Apportion: To distribute or allocate something, in this case, shots, among different players.
Approximation: A close estimate or representation.
Arbitrary: Based on random choice or personal preference, rather than any reason or system.
Beatdown: A slang term used to describe a decisive and one-sided victory or defeat.
Centralization: The degree to which something is concentrated or focused around a few key elements or individuals.
Coherent defensive structure: A well-organized and coordinated defensive system in basketball, where players work together to protect their own basket and prevent the opposing team from scoring.
Connections (edges): The links or relationships between different elements (nodes) in a network.
Connectivity: The extent to which elements in a network are connected or able to communicate with each other.
Constant: Something that remains the same or does not change.
Contingency plans: Plans or strategies prepared in advance to deal with potential unexpected events or situations.
Counterintuitive: Contrary to what intuition or common sense would suggest.
Data: Facts or information collected for analysis or reference.
Decentralized: The opposite of centralized, refers to a situation where something is spread out or distributed among multiple elements or individuals.
Decline: To decrease or become less in amount, quality, or intensity.
Denominator: The bottom part of a fraction.
Digraph: Short for "directed graph," it refers to a network or graph where the connections between nodes have a specific direction or flow.
Directed network: A type of network where the connections or edges between nodes have a specific direction or flow.
Distribution of decision-making: How the responsibility for making decisions is spread or allocated among different individuals or nodes in a network.
Dynamics: The patterns or changes in behavior over time within a system.
Edges directed: Refers to the connections or links between elements in a network that have a specific direction or flow.
Edges: The lines or connections between vertices in a network.
Efficacy: The effectiveness or efficiency of something.
Efficiency: The ability to accomplish something with the least waste of time, effort, or resources.
Elements (nodes): The individual components or units within a network.
Equilibrium: A state of balance or stability where opposing forces or factors are equal.
Evidently: Clearly or obviously.
Ewing Theory: A sports theory named after basketball player Patrick Ewing, suggesting that a team might perform better when their star player is absent or injured.
Extent: The degree to which something happens or is true.
Extracted: Obtained or derived from a source.
Foregone conclusion: An outcome that is predictable or inevitable.
Hypothetical: Based on or serving as a hypothesis or assumption.
Incentive: A motivating factor or reason that encourages someone to do something.
Incentive: Something that motivates or encourages someone to do something.
Incorporation: The act of including or combining something as a part of a whole.
Inordinate: Excessive or unusually large in amount or degree.
Intently: With earnest and eager attention.
Inverse relationship: A relationship between two variables in which one variable increases as the other decreases, and vice versa.
Key: Important or essential.
Mathematical framework: A structure or system of mathematical principles used to analyze or understand a particular concept or phenomenon.
Model: A simplified representation or description of a system or concept used to study or understand its behavior or properties.
Nash equilibrium: A concept in game theory where no player can improve their situation by unilaterally changing their strategy while the other players keep their strategies unchanged.
Network: A system of interconnected elements or people.
Network approach: The method or perspective of analyzing and understanding basketball offense (or any other system) as a network of interconnected elements and relationships.
Network diagram: A visual representation or drawing that shows the connections and relationships between elements in a network.
Network theory: A mathematical framework that studies the connections between elements within a network.
nodes: In the context of networks, they represent the individual elements or entities within the network. In this passage, players are referred to as nodes.
Numerator: The top part of a fraction.
Optimal: The best or most favorable result or solution.
Optimal pathfinding: Algorithms or methods used to find the most efficient or best route from one point (node) to another in a network.
Paradox: A statement or situation that seems contradictory or opposed to common sense but may be true.
Pathway: The route or sequence of movements, actions, or connections between nodes in a network.
Penciling in: Making a tentative or provisional plan or prediction.
Phenomenon: A fact or event that can be observed or experienced.
Plot: A graph or diagram that shows the relationship between variables.
Price of anarchy: A measure of the inefficiency in a system when individuals act in their self-interest, resulting in a suboptimal outcome for the group.
Real-world systems: Actual systems or phenomena that exist in the world, outside of a theoretical or abstract context.
Reg. season: Short for "regular season," referring to the scheduled games in a sports league before the playoffs.
Regression: A statistical technique used to analyze the relationship between variables and predict future outcomes.
Resilience: The ability of something to withstand or recover from external disturbances or disruptions and continue to function effectively.
Sacrifice: To give up something valuable or important for the sake of something else.
Simplification: The act of reducing something to its essential or basic features for easier understanding.
Simultaneously: At the same time.
Skill curve: A theoretical concept in basketball suggesting that there is a relationship between a player's offensive usage (how often they shoot) and their offensive efficiency (how successful their shots are).
Spatial relationships: The connections or interactions between elements in a physical space.
Strategy: A plan of action designed to achieve a particular goal.
Sub-optimality: A state or condition that is less than optimal or less than the best possible.
True shooting percentage: A basketball statistic that measures a player's shooting efficiency, taking into account field goals, free throws, and three-point shots.
Undirected network: A type of network where the connections or edges between nodes do not have a specific direction.
Vertices: The points or locations where lines or edges intersect in a network.
Weighted graph: A graph where the connections or edges between nodes have assigned weights or values to represent the strength of the interaction or relationship.
The Price of Anarchy in Basketball
By Brian Skinner, Journal of Quantitative Analysis in Sports - Vol. 6, #1, 2010, Article 3
(abridged & augmented by David Trotman)
Basketball can be viewed as a network problem:
-
Each possession has a definite starting point (the sideline or baseline in-bounds pass) and a definite goal (putting the ball in the basket).
-
Each possession takes place through a particular pathway: the sequence of player movements and passes leading up to the shot attempt.
-
Players are nodes, and passes between them are paths. Each position (Point Guard, Shooting Guard, Small Forward, Power Forward, and Center) is his own specific node.

When a coach diagrams a play for his/her players, he/she is essentially instructing them to move the ball through a particular pathway in order to reach the basket. If we think of a basketball offense as a network of possibilities for moving from in-bounds pass to basket, then we must imagine each pathway to have a different efficiency that [in part] depends on its frequency of use.
Imagine a simple give-and-go play between the and the Point Guard and the Power Forward. It could be expressed as a simplified pathway like this:
(Point Guard) pass −→ (Power Forward) pass −→ (Point Guard) shot −→ (basket)
Each arrow represents a movement of the ball, beginning in the hands and ending in the basket. The efficiency of this particular play (its likelihood of producing points) will be unique, and different from all other pathways such as the variation,
(Point Guard) pass −→ (Power Forward) pass −→ (Center) layup −→ (basket).
The efficiency of a play should also depend on the extent to which it is used. The give-and-go, for example, may be very effective when seldomly used, but if the team tries to run it every time down the court then the defense will learn to anticipate their opponents’ moves and the offense will find much less success.
Even if the complementary “network” of defensive rotations and adjustments is not considered explicitly, there are still five interacting offensive players and an infinite set of potential plays and broken plays to be accounted for. The network approach can provide valuable insight into the operations and inefficiencies of a basketball offense. In particular, the idea of a “price of anarchy” may provide a useful definition for measuring the difference between a team’s operating efficiency and its maximum possible production.
The Price of Anarchy on a Highway
Two points A and B connected by a pair of parallel roads. Imagine that ten cars have to get from point A to point B, and that connecting A and B are two roads.

The upper road (labeled 1) is a wide highway. It takes ten minutes to travel regardless of the number of cars on it. In other words, the length of time (L1) of a driver’s commute on the highway is constant, and where x1 indicates a vehicle L1(x1) = 10 minutes. L1(x1) in words is; the time it takes for each car to go on the highway from A to B is 10 minutes, and for purposes of this example, no matter how many cars are on the highway it is going to take 10 minutes to go from A to B.
The lower road (labeled 2) is a narrow alley. It is more direct but cannot hold much traffic without becoming significantly slower. Say that the length of time it takes commute along the alley depends on the number of cars x2 that take it as L2(x2) = x2 minutes. That is, if only one car takes the alley, then that car has a 1-minute commute. If two cars take the alley, then they each have a 2-minute commute, and so on. Evidently, there is no reason for anyone to take the highway. At worst, driving through the alley will take ten minutes, while the highway always takes ten minutes. It is therefore in the best interest of all ten drivers to take the alley. This situation results in an average commute time (Li)NE = 10, and is called a “Nash equilibrium”.
In general, the Nash equilibrium is the state where no individual can improve his situation by making a different choice. In this particular case, it is the state where each driver has the same length of commute.
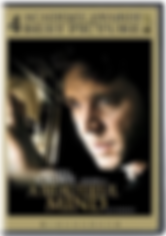
John Nash, the man who gave his name to the Nash equilibrium won the 1994 Nobel Prize for Economics. A film, “A Beautiful Mind” was made based on his academic achievements and subsequent psychological problems. In 2002 it won the Academy Award for Best Picture.
For the drivers in this example, the Nash equilibrium is not actually the best possible outcome. For arbitrary usage levels X1, X (2) of the two roads, the average commute length is

Ellis left Xerox PARC in the mid-1980s to lead the Groupware Research Group at the Microelectronics and Computer Technology Corporation (MCC). While at MCC, he led efforts in Real-time Collaborative Editing, and Operational Transformation.
The above equation is much scarier looking than it actually is. In words, on the left side it says that the numerator is length of time on road one times the number of cars plus the number of cars on road two times the length of the journey divided by the denominator which is the total number of cars. On the right side it says the number of cars on road one (remember the journey time is constant on road one) plus the number of cars on road two squared divided by 10.
Since there are ten cars total, X1 and X2 satisfy X1+X2 = 10, and by substitution (this substitution represent drivers choosing the alley alternative).
We have hLi= X1 + (10−X1)2/10. (2)
What we want to find is the arrangement where the total time for all drivers is minimalized. Then is this different than the Nash equilibrium, where no driver may decrease his travel time.
[Note: Though this can be done through calculus, as an intro lesson through a repetitive arithmetic process the minimum will be identified. - DT]
The optimum scenario has 5 cars getting 10-minute commutes and 5 cars getting 5-minute commutes, while at the Nash equilibrium all 10 cars had 10-minute commutes. This optimum is not stable. Each of the 5 people driving on the highway has an incentive to switch to the alley, whereby he/she could reduce his/her driving time from 10 minutes to 6. So if the global optimum is to be maintained, there must be some kind of structure enforced upon the drivers. That is, the drivers must be “coached” into the global optimum, because it is against their immediate best interest. The difference between the Nash equilibrium and the real optimum is called the “price of anarchy.” Here it is
hLiNE −hLiopt = 2.5 minutes, or 33% when ((hLiNE−hLiopt)/ hLiopt)
If more traffic complicated networks are considered, then the discrepancy between the Nash equilibrium and the global optimum can allow for counterintuitive (the word counterintuitive is a key concept for understanding one of the reasons why mathematics is really useful) consequences
In terms of the global optimum, closing a road must always have a negative effect; it removes potential options whereby traffic may flow. However, closing a road can have the effect of greatly disturbing the Nash equilibrium, and perhaps pushing it closer toward the global optimum distribution. Since real-world traffic follows something much closer to the Nash equilibrium than the global optimum, it is fully possible that closing down a road can improve traffic. This phenomenon is called “Braess’s Paradox”, and it has apparently been observed in cities like New York, San Francisco, and
Stuttgart, where the closing of a major road was accompanied by almost immediate improvements in traffic flow.
In sports, ESPN.com sportswriter/humorist Bill Simmons (2001) called “Braess’s Paradox”, the “Ewing Theory.” The idea that a team could improve after losing one of its best players may in fact have a network-based justification, and not just a psychological one. That is, one can easily imagine a network of plays whose Nash equilibrium will improve when a key player is removed.
Ewing Theory 101
By Bill Simmons (edited & augmented by David Trotman)
You're probably tired of reading those "Where did these guys come from?" stories about the Seattle Mariners, who valiantly clawed their way to baseball's best record [For the Seattle Mariners' 2001 season they achieved a record of 116–46, tying the Major League record for wins set by the Chicago Cubs in 1906 and setting the record for wins by an American League team.] earlier this season, despite losing all-stars Randy Johnson, Ken Griffey Jr. and Alex Rodriguez over the previous three years.
How can a franchise prosper after losing three of the biggest stars in baseball? How does this make sense?
I have a three-word explanation for you: "The Ewing Theory."
The theory was created in the mid-'90s by Dave Cirilli. [He] was convinced that Patrick Ewing's teams (both at Georgetown and with New York) inexplicably played better when Ewing was either injured or missing extended stretches because of foul trouble.
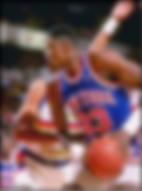
Patrick Ewing
Curious to see if this phenomenon applied to other stars/teams, Dave noticed people were penciling in the '94-'95 UConn Huskies for a .500 season because "superstar" Donyell Marshall had departed for the NBA. Dave knew better; a lifelong UConn fan, he thought the Huskies relied too much on Marshall the previous season and could survive without him. Dave told friends the Huskies would thrive in Marshall's absence -- and that's exactly what happened. By midseason, UConn was ranked No. 1 in the country for the first time in school history; the Ewing Theory had been hatched.
Dave introduced me to the Ewing Theory years ago, and [since then], we've been tinkering with it. Eventually, we decided that two crucial elements needed to be in place for any situation to qualify for "Ewing" status:
1. A star athlete receives an inordinate amount of media attention and fan interest, and yet his teams never win anything substantial with him (other than maybe some early-round playoff series).
2. That same athlete leaves his team (either by injury, trade, graduation, free agency or retirement) -- and both the media and fans immediately write off the team for the following season.
What's the best example of the Ewing Theory?
During the '99 NBA Playoffs, Ewing tore an Achilles tendon during the second game of the Eastern finals against Indiana. With Ewing finished for the playoffs and nobody else on the Knicks who could handle [Indiana Center] Rik Smits, the series seemed like a foregone conclusion. As an added bonus, since Ewing himself was involved, that made this the ultimate test of the Ewing Theory; in fact, I e-mailed Dave that week to say, "This is the greatest test yet."
Dave…oozed with confidence, as he told me in no uncertain terms, "Ewing's injury is the best thing that ever could have happened to the Knicks -- they're definitely making the Finals now."
So what happened? The Knicks won three of the next four and advanced to the NBA Finals for only the second time in 26 years. Had Jeff Van Gundy's crew shocked the Spurs in the Finals without Ewing, Dave might have his own line of "How-To" videos out right now (a Knicks upset was simply too tall of a task against [Tim] Duncan and [David] Robinson, Ewing Theory or no Ewing Theory).
Braess’ Paradox: The Washington Wizards & All-Star Guard John Wall

“Just look at the team’s record. The Wizards were 26-22 (26 wins, 22 losses) when Wall went down with a knee injury in January. Since then, the team is 11-6 with backup guard Tomas Satoransky taking most of Wall’s minutes.” -https://www.washingtonpost.com/lifestyle/kidspost/ when-john-wall-was-injured-the-wizards-changed-their-game-will-he-change-too/2018/03/07/ 33799c38-17f0-11e8-92c9-376b4fe57ff7_story.html
[The Wizards finished the 2017-18 NBA season with 43-39 record. With Wall in the line-up, their record was 28-27. With Wall out of the line-up their record was 15-12. On the basis of this limited information, it would seem that Wall’s presence is neither a major positive nor a negative for the team. Given his all-star status and commensurate salary, this tentative conclusion appears to be somewhat counterintuitive and a bit supportive of the “Ewing Theory”. - DT]
Braess’ Paradox: The Memphis Grizzlies & All-Star Guard Ja Morant

“The Grizzlies have a 21-6 record (.777) without Ja Morant this year (reg. season & part. playoffs), and have just completed a 39-point beatdown of Golden State without their All-Star PG.” - Peter Edmiston
[This would seem to support the “Ewing Theory”. - DT]
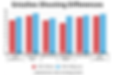
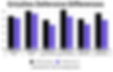
On the basketball court, possessions are like cars. Each one starts at point A (the in-bounds) and attempts to travel to point B (the basket). Different plays are like different roads: each one has a different efficiency that will generally decrease the more it is used. It may seem like common sense to say that every play in a basketball game should be run in the way that gives it the highest probability of success. Such a strategy is like the “selfish” Nash equilibrium, and it is not necessarily the one the leads to the highest overall efficiency. A team that contents itself with making the highest-percentage play each time down the court and does not consider the game-wide implications of its strategy can pay a significant price of anarchy for its “short-sighted” approach.
A simplified offensive for a basketball offense: it should look something like Fig. 2. Each line connecting the beginning of the possession to the shot attempt represents a different player, who is treated as a possible path by which points may come. Each player (labeled i = 1, 2,..., 5) has a particular scoring efficiency (fi) that depends on his frequency of use(xi).

Figure 2: Five paths by which a team may acquire points.
Each path represents a shot attempt by one of the team’s five players.
Fig. 2 is a drastic simplification of the beautiful complexities of a real offense: it assumes that the only thing relevant for the efficiency of a shot is who takes it. In reality, the efficiency of a shot should depend on the play leading up to it, but this simplified model may nonetheless be instructive as a first attempt.
The “efficiency of a shot” is something like “probability that a shot produces points”. While field goal percentage would be a close approximation, a slightly more advanced statistic has already been invented for this concept. It is called “true shooting percentage”, or TS%, and is best thought of as a field goal percentage adjusted for free throws and three-point shots.

The efficiency function fi(xi) of a player can therefore be defined as the player’s TS% as a function of the fraction of the team’s shots xi that he takes while on the court.
In “Basketball on Paper” (2004), Dean Oliver envisioned such an inverse relationship between offensive usage and offensive efficiency. He dubbed it a “skill curve”, although he used somewhat different definitions of “usage” and “efficiency” than are employed here. The skill curve remains a highly theoretical object that has never been extracted from data in a generally accepted way. Nonetheless, something approximating a skill curve can be estimated by examining season averages for TS% and shot volume among players whose offensive load has varied significantly over the course of their playing career. This approach assumes that the league as a whole maintains a similar quality of defense from year to year, so that the skill curve is defined in terms of the average defender that the player is likely to encounter
Consider the season averages for former NBA all-star, Boston Celtics guard Ray Allen.

Fig. 3 shows a plot of Allen’s TS% as a function of the fraction of his team’s shots x that he took while on the court; the solid line is a linear best-fit. The first three seasons of his career (open circles) were not included in the fit lines, as this was considered a “learning curve” period wherein Allen was still improving.

Figure 3: Estimated “skill curve”
Each data point represents the average performance over a given season of his professional career. The solid line is a linear fit, shown with its R2 (regression) correlation value. Open circles represent the first three seasons of his career, and are not included in the fit line.
The fraction x was calculated as

The existence of a negative relationship between shot volume and shot efficiency seems at least plausible. It is reasonable to assume that, over the relevant range of shot fraction x, the function f(x) is linear. Further analysis will use this assumption.
Equilibrium versus Optimum
Suppose that a team of five players is aware of each other’s skill curves. How should they distribute their shots in order to achieve optimal efficiency? The most obvious strategy is to always give the ball to the player with the highest percentage chance of making the shot. That is, if Player A is shooting with a higher TS% than Player B, this strategy calls for the team to apportion more shots to Player A, which process continues until player A’s efficiency declines to the level of player B’s (or Player B’s efficiency rises to the level of Player A’s). The result is to establish a kind of equilibrium among all five players, such that all five shoot the same percentage.
In other words, the strategy of looking for the highest percentage option each time down the court results in an equilibrium defined by
f1(x1) = f2(x2) = ... = f5(x5)
All that is going on here is efficiency of each player (the likelihood of their shot going in) depends on the number of shots they take, and ideally each should take the when its likely to go in.
The analogy with the Nash equilibrium in the traffic example is straightforward. In traffic, the Nash equilibrium was reached when each driver took the path that was in his/her best interest. The equilibrium of f1(x1) = f2(x2) = ... = f5(x5) is reached when each possession follows the path that is in its best interest. The Nash equilibrium is not necessarily the team’s best strategy. The true best strategy will be the one that optimizes the team’s overall efficiency F, defined as
F = x1f(x1) + x2f(x2) + ... + x5f(x5)
If the team’s efficiency when it follows the optimum strategy is denoted as Fopt, and its efficiency when it follows the Nash equilibrium is denoted FNE, then the price of anarchy for the offense is defined as Fopt −FNE.
To illustrate the significance of the optimum strategy, and the extent to which it can differ from the Nash equilibrium, we can consider that Ray Allen is on a team with four “lesser” players, who generally have a lower TS% than he does. The plot in Fig. 3 suggests that Ray Allen’s TS% is fRA(x)≈0.75 - 0.62x Imagine now that Allen’s teammates shoot with a constant efficiency f = 0.5, regardless of their shot volume (which will never be larger than 25% each, anyway). In this scenario, Ray Allen is like the “alley”, which provides a more efficient path to the goal but declines with usage, while Allen’s teammates are like the highway.
If Ray Allen were not playing like the “highway”, which is less efficient but does not decline in efficiency with usage, the Nash equilibrium strategy would require that Ray Allen continue shooting until he is only as efficient as his teammates: fRA(xNE) = 0.5. Consequently, Ray Allen would take xNE ≈0.40 of his team’s shots and the team would have a TS% of FNE = 0.5. In this extreme case, it is probably clear that the Nash equilibrium is not the optimal strategy. A team with Ray Allen, who is an exceptional player, should not shoot only as well as his less-than-exceptional teammates. More generally, if Ray Allen shoots some fraction x of his team’s shots, then the team’s TS% will be F = xfRA(x) + (1−x) × 0.5. This result is fairly surprising. It suggests that the team’s optimum strategy is for Ray Allen to shoot almost exactly the same fraction of shots as his teammates — about 20%. In such a scenario Allen would have a TS% of 0.625, far above the rest of his teammates who are shooting with a TS% of 0.5. It would seem to be obvious that Ray Allen should be getting more shots than his teammates. But the result of limiting Allen’s shots, and thereby keeping the defense from focusing too intently on him, pays off. In this case it improves the team’s TS% by 2.5%. For reference, in the 2008-2009 NBA season the standard deviation among teams in TS% was only 1.6%, so a difference of 2.5% may be quite significant. To achieve it, the team just needs to consciously choose not to have Ray Allen shoot on ∼80% of possessions, even when he is their best option.
If Ray Allen’s team is playing by the Nash equilibrium, then it doesn’t make a difference whether he is in the game or not. When Ray Allen plays, the Nash equilibrium settles at a team TS% of 0.5. But the team would shoot this same percentage even if Ray Allen did not take a single shot. So this hypothetical team, if playing by the Nash equilibrium and not with the optimal strategy, would lose nothing if Ray Allen sat out.
Consider, for example, the network diagrammed in Fig. 5, which is designed to produce a layup for the Point Guard (labeled 1), the Shooting Guard (2), or the Center (5). The play starts with the ball in the hands of either PG (1) or SG (2), who drives from the top of the key toward the basket. When he arrives at the low post, the driver has two options: he can put up a shot at the basket or he can shovel the ball to the (5).

Figure 5: A dribble penetration play, which starts with either the Point Guard (1) or the Shooting Guard (2) driving to the basket, and ends with a shot by one of the guards or the Center (5).
If the Center receives the ball, he also has two options: he can take a shot or he can pass the ball to a cutting PG or SG— whichever guard it was that did not make the initial drive to the basket — for the layup. Fig. 5 shows this network of possible ball movements. Solid lines indicate a drive or shot attempt, and dashed lines represent passes. Next to each solid line is a formula that is meant to show the effectiveness of that step of the play, i.e. the probability that the given step will be completed successfully. Here, x stands for the fraction of the time that the given step is used; x = 1 if the ball always proceeds through this route and x = 0 if the route is never used. The choice of efficiencies here is arbitrary, but the situation can be imagined like this: The Point Guard (1) is a quick and capable ball handler, but is mediocre at finishing around the rim. The Shooting Guard (2) is not particularly good at driving the ball, but is significantly better at finishing around the basket. The Center (5) is somewhere in the middle as a shooter: not as good as the SG, but better than the PG. For simplicity, it is assumed that all passes are successful every time. The Nash equilibrium can be easily calculated for this network once the following observations are made:
-
The SG (2) is never more successful at driving the ball than PG (1). Even at his very worst, PG (1) is successful on 50% of his drives while SG (2) is always 50% successful.

Figure 6: The same dribble penetration play as in Fig. 5, but with the Center (5) removed from the play.
PG (1) is never more successful at shooting than SG (2) or C (5). At their very worst, SG (2) and C (5) are 50% effective at finishing at the rim while PG (1) always shoots 50%.
By the Nash equilibrium strategy, where the effectiveness of each individual possession is optimized, there is apparently no reason for SG (2) to ever drive or for PG (1) to ever shoot. That is, PG (1) should drive the ball every time and then pass to the Center. The only remaining question is how often C (5) should attempt the layup himself and how often he should pass to SG (2) for the finish. In the Nash equilibrium strategy, this question is also easily answered by equating the shooting percentages of C (5) and SG (2). The result is for C (5) to take the shot 1/3 of the time and SG (2) to take the shot 2/3 of the time. Both players make the layup 2/3 of the time. The overall efficiency of the play is therefore 0.5 × (2/3) = 0.33. We can now consider how the play might look if C (5) is removed from the offense — perhaps the original player is lost to injury and a replacement is introduced who does not get involved in the offense, but merely stands off to the side when the play is run. In this situation, all passes to or from C (5) are eliminated, and the only options for the play are a drive and shot by PG (1) or a drive and shot by SG (2). So the offensive network is simplified to the one depicted in Fig. 6. In this case, the two guards PG (1) and SG (2) constitute equivalently effective options for the play — PG (1) is good at the drive and worse at the shot while SG (2) is good at the shot and worse at the drive, so that their overall efficiencies in running the play from the top of the key are equal. As a consequence, the guards PG (1) and SG (2) split their shot attempts 50/50, and each scores with an efficiency 0.5×(1−0.5×0.5) = 0.375. This is the same as the overall efficiency of the play, and, surprisingly, it is higher than the 0.33 efficiency that resulted when the Center was involved. This counterintuitive result is completely analogous to Braess’s Paradox, an important component of the network is removed, but the overall efficiency improves. It is worth noting that in the original play, run at the Nash equilibrium, the Center was an absolutely key contributor. He touched the ball every time the play was run and shot 1/3 of the time with a 67% success rate. A team that found its success improved after losing such a player would likely be extremely surprised. Of course, this result really highlights the sub-optimality of the “shortsighted” Nash equilibrium solution. The true optimum performance for this offense can be found not by considering the best way to run each individual play, but by considering every option simultaneously and optimizing the total performance with respect to the frequency that each option is run.
The optimum strategy involves PG (1) driving 33% of the time and SG (2) driving 67% of the time. As for the shot attempt, PG (1) should shoot 5% of the time, SG (2) should shoot 45% of the time, and C (5) should shoot 50% of the time, even though in this case the three players will shoot very different percentages. The resulting overall efficiency of the play is 0.42, well above the Nash equilibrium solution 0.33 or the efficiency 0.375 that resulted when C (5) was removed. In other words, there is a significant price of anarchy for this offensive set. When run with the best possible strategy, the play can be successful 42% of the time. But when the team only considers one possession at a time, the play works only 33% of the time. The apparent improvement of the team upon losing its Center, and, indeed, any instance of the “Ewing Theory”, is a consequence of this short-sightedness.
Conclusions
The defense has been treated only implicitly in this approach. In principle, a defense should be able to choose to focus on a given player to an arbitrary degree, thus shifting that player’s skill curve up or down. It may be that the full incorporation of a defense’s “right to choose” will weaken the conclusions. Nonetheless, the idea of a difference between Nash-like equilibrium states and a global optimum strategy is enticing. If a team could be fully aware of the efficiencies of all of its plays and players (its “skill curves”), then it could easily determine the exact best way to allocate shots, passes, drives, etc. and could quantify the difference between its current operating efficiency and its maximum potential.
A basketball coach calling a play for his team is probably aware that he/she should not always simply call the play with the highest probability of success. Rather, a coach may decide to “save” the team’s best plays for later, with the purpose of retaining their high efficiency against the defense. In fact, this kind of reasoning is almost certainly in the minds of coaches and players in other sports. In baseball, a pitcher will often decide not to throw his best pitch — even if it means a higher chance of giving up a hit in the short term — so that the batter is less prepared when he does use his “best stuff”. In American football, every professional team produces more yards with the average passing play than with the average running play. Nonetheless, teams continue to employ less efficient running plays as a means of “keeping the defense honest”. That is, the immediate success of a given play is sacrificed in order to maintain the high efficiency of a team’s offense as a whole.
STEM Topic 26: Problem Set
-
In the following examples, work out how many “Vertices”, “Edges”, and “Regions” there are. Remember to count the area outside each Network as a Region. Network Equation: R + V = E + 2


NETWORK TOPOLOGY TYPES




2. In a star network topology, there are 8 devices connected to a central switch. Each device has 4 ports. How many total connections are there in the network?
3. In a ring network topology, there are 12 devices connected in a circular manner. Each device has 2 ports. How many total connections are there in the network?
4. In a bus network topology, there are 6 devices connected in a linear manner. Each device has 2 ports. How many total connections are there in the network?
5. In a mesh network topology, there are 7 devices connected in a fully connected manner. Each device has 6 ports. How many total connections are there in the network?
6. In a tree network topology, there are 5 devices connected in a hierarchical manner. The root device has 3 ports, and each subsequent level has twice the number of ports as the previous level. How many total connections are there in the network?
7. In a star network topology, there are 10 devices connected to a central switch. If each device has 5 ports, and each port can be connected to one other device, how many total connections are there in the network?
8. In a bus network topology, there are 8 devices connected in a linear manner. Each device has 3 ports. If two devices are added to the network, how many total connections will there be?
9. In a ring network topology, there are n devices connected in a circular manner. Each device has 2 ports. If n = 9, how many total connections are there in the network?
10. In a mesh network topology, there are 6 devices connected in a fully connected manner. Each device has n ports. If the total number of connections is 30, what is the value of n?
11. In a tree network topology, there are 4 devices connected in a hierarchical manner. The root device has 2 ports, and each subsequent level has one less port than the previous level. How many total connections are there in the network?
12. In a star network topology, there are 15 devices connected to a central switch. Each device has 3 ports. If 5 devices are removed from the network, how many total connections will there be?